ABELARDO MONTESINOS LÓPEZ
Resumen curricular:
Abelardo Montesinos López es Licenciado en Estadística por la Universidad Autónoma Chapingo, Maestro y Doctor en Probabilidad y Estadística por el... Ver más
Abelardo Montesinos López es Licenciado en Estadística por la Universidad Autónoma Chapingo, Maestro y Doctor en Probabilidad y Estadística por el Centro de Investigación en Matemáticas (CIMAT), Guanajuato, México. Actualmente es profesor en el departamento de Matemáticas (CUCEI), Universidad de Guadalajara. Miembro del Sistema Nacional de Investigadores Nivel I. Cuenta con más de 50 artículos y es coautor de 2 libros y de más de 5 capítulos de libros. Entre sus áreas de interés están el desarrollo y/o aplicación de modelos de predicción genómica para fitomejoramiento, modelos mixtos lineales generalizados, análisis de supervivencia, análisis bayesiano y análisis multivariado. Ver menos
Perfil de Investigador SNII:
Perfil PRODEP:
Cuerpos académicos:
Estadística
Bases de datos bibliográficas:
Scopus
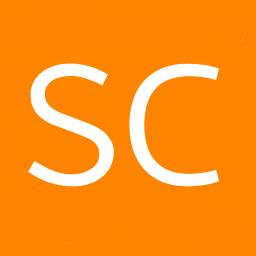
ORCID
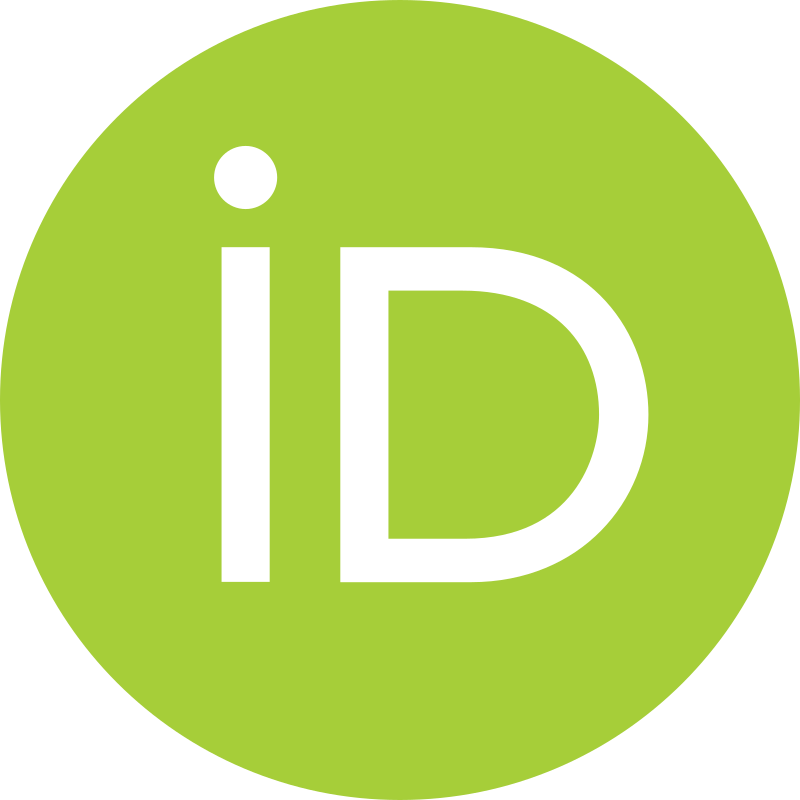
Publicaciones del académico:
- Data Augmentation Enhances Plant-Genomic-Enabled Predictions
- A marker weighting approach for enhancing within-family accuracy in genomic prediction
- Bayesian discrete lognormal regression model for genomic prediction
- Deep learning methods improve genomic prediction of wheat breeding
- Partial least squares enhance multi-trait genomic prediction of potato cultivars in new environments
- Two simple methods to improve the accuracy of the genomic selection methodology
- Designing optimal training sets for genomic prediction using adversarial validation with probit regression
- Multivariate Genomic Hybrid Prediction with Kernels and Parental Information
- Efficacy of plant breeding using genomic information
- Sparse multi-trait genomic prediction under balanced incomplete block design
- Statistical Machine-Learning Methods for Genomic Prediction Using the SKM Library
- Multimodal deep learning methods enhance genomic prediction of wheat breeding
- Optimizing Sparse Testing for Genomic Prediction of Plant Breeding Crops
- Integrating Parental Phenotypic Data Enhances Prediction Accuracy of Hybrids in Wheat Traits
- A novel method for genomic-enabled prediction of cultivars in new environments
- Do feature selection methods for selecting environmental covariables enhance genomic prediction accuracy?
- A Multi-Trait Gaussian Kernel Genomic Prediction Model under Three Tunning Strategies
- A Comparison between Three Tuning Strategies for Gaussian Kernels in the Context of Univariate Genomic Prediction
- Multi-trait genome prediction of new environments with partial least squares
- A Comparison of Three Machine Learning Methods for Multivariate Genomic Prediction Using the Sparse Kernels Method (SKM) Library
- Partial Least Squares Enhances Genomic Prediction of New Environments
- A General-Purpose Machine Learning R Library for Sparse Kernels Methods With an Application for Genome-Based Prediction
- Using an incomplete block design to allocate lines to environments improves sparse genome-based prediction in plant breeding
- Bayesian multitrait kernel methods improve multienvironment genome-based prediction
- A Revision of the Traditional Analysis Method of Allometry to Allow Extension of the Normality-Borne Complexity of Error Structure: Examining the Adequacy of a Normal-Mixture Distribution-Driven Error Term
- Comparing gradient boosting machine and Bayesian threshold BLUP for genome-based prediction of categorical traits in wheat breeding
- Methods in Molecular Biology
- Accounting for Correlation Between Traits in Genomic Prediction
- Genome and Environment Based Prediction Models and Methods of Complex Traits Incorporating Genotype × Environment Interaction
- Plant Genotype to Phenotype Prediction Using Machine Learning
- A New Deep Learning Calibration Method Enhances Genome-Based Prediction of Continuous Crop Traits
- A review of deep learning applications for genomic selection
- Application of a Poisson deep neural network model for the prediction of count data in genome-based prediction
- Deep-learning power and perspectives for genomic selection
- Multi-trait genomic-enabled prediction enhances accuracy in multi-year wheat breeding trials
- The Modern Plant Breeding Triangle: Optimizing the Use of Genomics, Phenomics, and Enviromics Data
- A guide for kernel generalized regression methods for genomic-enabled prediction
- A zero altered Poisson random forest model for genomic-enabled prediction
- A multivariate poisson deep learning model for genomic prediction of count data
- Maximum a posteriori threshold genomic prediction model for ordinal traits
- Genome-based prediction of multiple wheat quality traits in multiple years
- Bayesian regularized quantile regression: A robust alternative for genome-based prediction of skewed data
- Maximum <i>a posteriori</i> Threshold Genomic Prediction Model for Ordinal Traits
- Multi-Trait, Multi-Environment Genomic Prediction of Durum Wheat With Genomic Best Linear Unbiased Predictor and Deep Learning Methods
- Modeling genotype × environment interaction using a factor analytic model of on-farm wheat trials in the Yaqui valley of Mexico
- Multivariate bayesian analysis of on-farm trials with multiple-trait and multiple-environment data
- A Bayesian genomic multi-output regressor stacking model for predicting multi-trait multi-environment plant breeding data
- Deep Kernel for genomic and near infrared predictions in multi-environment breeding trials
- An R package for Bayesian analysis of multi-environment and multi-trait multi-environment data for genome-based prediction
- New deep learning genomic-based prediction model for multiple traits with binary, ordinal, and continuous phenotypes